ResearchComputing with neuronal cliques
What is the right abstraction for biological neural networks? While much of the machine learning community is concentrating on feedforward architectures, there is a rich set of feedback connections as well. What can be solved in networks with lateral connections within an area and feedforward/feedback connections between areas? Can optimization and inference problems be solved with only a few spikes?
We discovered that it is possible to solve a family of these problems with only a few spikes in 10s of msecs, provided the neurons are organized into (self-excitatory) cliques:
- Computing with Self-Excitatory Cliques: A Model and An Application to Hyperacuity-scale Computation in Visual CortexNeural Computation11121-661999
It follows from the theoretical observation that the network equations are related to polymatrix games:
- Efficent simplex-like methods for equilibria of nonsymmetric analog networksNeural Computation42167 - 1901992
...which in turn build upon relaxation labeling, our early mechanism to enforce consistency (note that "Hopfield Energy" is called "Average Local Consistency"):
- On the foundations of relaxation labeling processesReadings in Computer Vision1987
Compatibility relationships are embodied in neuronal connections, and these can be developed directly from geometric considerations:
- Geometrical computations explain projection patterns of long-range horizontal connections in visual cortexNeural Computation163445 - 4762003
For a more recent review, see also:
- Stereo, Shading, and Surfaces: Curvature constraints couple neural computationsProc. IEEE1025812-8292014
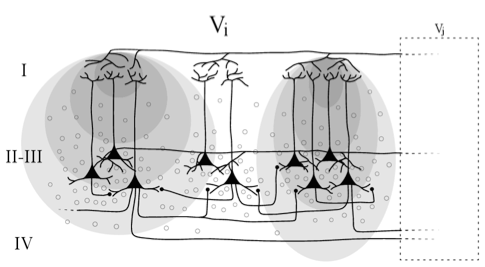