Research
You can download a copy of my research statement or a detailed CV.
Human
behavior has been studied from many perspectives and at many
scales. Psychology, sociology, anthropology, and neuroscience
each use different methodologies, scope, and evaluation criteria to
understand aspects of human behavior. Computer science, and in
particular robotics, offers a complementary perspective on the study of
human behavior.
My research focuses on building embodied
computational models of human social behavior, especially the
developmental progression of early social skills. My work uses
computational modeling and socially interactive robots in three
methodological roles to explore questions about social development that
are difficult or impossible to assail using methods of other
disciplines:
- We explore the boundaries of human social abilities by studying human-robot interaction.
Social robots operate at the boundary of cognitive categories; they are
animate but are not alive, are responsive but are not creative or
flexible in their responses, and respond to social cues but cannot
maintain a deep social dialog. By systematically varying the
behavior of the robot, we can chart the range of human social
responses. Furthermore, because the behavior of the machine can
be precisely controlled, a robot offers a reliable and repeatable
stimulus.
- We model the development of social skills using a robot as an embodied, empirical testbed.
Social robots offer a modeling platform that not only can be repeatedly
validated and varied but also can include social interactions as part
of the modeled environment. By implementing a cognitive theory on
a robot, we ensure that the model is grounded in real-world
perceptions, accounts for the effects of embodiment, and is
appropriately integrated with other perceptual, motor, and cognitive
skills.
- We enhance the diagnosis and therapy of social deficits using socially assistive technology.
In our collaborations with the Yale Child Study Center, we have found
that robots that sense and respond to social cues provide a
quantitative, objective measurement of exactly those social abilities
which are deficient in individuals with autism. Furthermore, children
with autism show a profound and particular attachment to robots, an
effect that we have leveraged in therapy sessions.
To pursue this research, considerable challenges in building interactive
robots must be surmounted. These challenges are at the leading
edge of a fundamental shift that is occurring in robotics
research. Societal needs and economic opportunities are pushing
robots out of controlled settings and into our homes, schools, and
hospitals. As robots become increasingly integrated into these
settings, there is a critical need to engage untrained, naïve users in
ways that are comfortable and natural. My research provides a
structured approach to constructing robotic systems that elicit,
exploit, and respond to the natural behavior of untrained users.
Human-Robot Interaction Projects:
Effects of Embodiment: My
research characterizes the unique ways that people respond to
robots. We focus in particular on how interactions with real
robots differ from interactions with virtual agents. One of our
results demonstrated that adults are more likely to follow
unusual instructions (such as throwing textbooks into the trash) when
presented by a physically present robot than when presented by the same
robot on a television monitor. We have also studied how
children's interactions with social robots elicit explanations on
agency and the qualities required for life. Pictured at right is
one of the robots that we use in these studies, an upper-torso humanoid
robot named Nico. Nico was designed to match the body structure
of the average 1-year-old male child. This platform serves as
both an interaction stimulus and as a testbed platform for our models
of social development. |
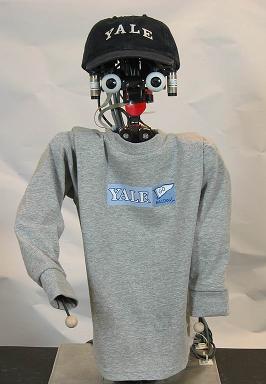 |
Prosody Recognition: If
speech recognition focuses on what you say, then prosody recognition
focuses on how you say it. Prosody is the rhythm, stress, and
intonation of speech and it provides an essential feedback signal
during social learning. Our research produced the first automatic
system for recognition of affect from prosody in male-produced speech,
the first demonstration that prosody can be used as feedback to guide
machine learning systems, and investigated the relation between prosody
and the introduction of new information in human-human conversations. |
Intention from Motion:
Humans naturally attribute roles and intentions to perceived agents,
even when presented with extremely simple cues. A human will watch
three animated boxes move around on a white background (as shown at
right), and describe a
scene involving tender lovers, brutal bullies, tense confrontations and
hair-raising escapes. We have constructed systems that classify
role and intent from the spatio-temporal trajectories of people and
objects, including systems that recognize who is "IT" during a game of
tag with performance that matches human judgments. Our future
work here focuses on how to link these low-level perceptual phenomena
with higher-level models of belief and goal-oriented behavior. |
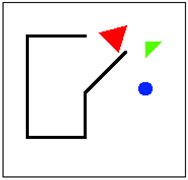 |
Computational Models of Social Development:
Joint reference:
We implemented a skill progression similar to that observed in children
in which the robot learns through unstructured interactions with an
untrained adult to first reach out and touch objects, then to point to
objects, and finally to follow the adult's direction of gaze to
identify objects of mutual interest (a behavior known as gaze following
or joint reference). This developmental progression acquires
higher accuracy than any other published system for joint reference and
requires 100 times fewer training examples. Furthermore, this
model provides a justification based on problem complexity that favors
active exploration and rich social engagement in human infants.
Pictured at right is the robot Nico (without its clothing)
engaged in one of these training sessions. The robot has selected
an object on the table, and the human observer has focused on that same
object without further communication. |
 |
Self-other discrimination: One
early developing skill that children must acquire is the ability to
discriminate their own body from the bodies of others. We
pioneered a kinesthetic-visual matching model for this task. By
learning the relationship between its own motor activity and perceived
motion, Nico learned to discriminate its own arm from the movements of
others and from the movement of inanimate objects. Furthermore,
this model works under conditions when an appearance-based metric would
certainly fail. Shown at right is an image from the robot's
camera with a red overlay on things it judges to be animate (but not
itself) and a green overlay on moving things that it considers to be
part of itself. Notice that when presented with a mirror, the
robot judges
both its own arm and its mirror image to be part of itself. This
result is unique among computational systems and calls into question
some of the common ethological tests of self-awareness that utilize
mirror recognition. |
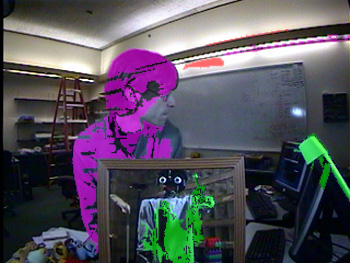 |
Language acquisition:
Most research on computational language acquisition focuses either on a
formal semantic model that lacks real-world grounding or on grounded
perceptual systems that acquire concrete nouns. We developed a
method for learning word meanings from real-world context that both
leverages formal semantic models and allows for a wide range of
syntactic types (pronouns, adjectives, adverbs, and nouns) to be
learned. We have constructed a system that uses sentence context
and its perceived environment to understand which object a new word
refers to, and that over time builds “definition trees” (shown at right) that allow both
recognition and production of appropriate sentences. For example,
in one experiment, Nico correctly learned the meanings for the words
“I” and “you,” learned that “this”
and “that” refer to objects of
varying proximity, and that “above” and “below” refer to height
differences between objects. This model provides a formal
computational method that explains both children's abilities to learn
complex word types concurrently with concrete nouns and explains
observed data on pronoun reversal. |
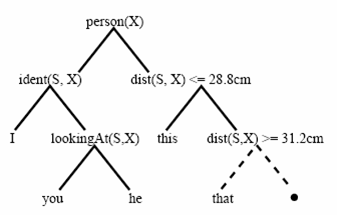 |
Diagnosis and Therapy for Social Deficits:
Quantitative metrics for autism diagnosis:
We have evaluated systems for prosody recognition, gaze
identification in unstructured settings, and motion estimation as
quantitative measurements for autism diagnosis. All of these techniques
address social cues that are deficient in many individuals with autism,
have previously been suggested as fundamental to diagnostic procedure,
and have been previously implemented on our robots. Our most
promising approach has been gaze tracking and parameter estimation
models of visual attention which has shown preliminary indications for
being an early screening tool for autism. We have shown a
pervasive pattern of inattention in autism differentiating 4 year old,
but not 2 year old, children with autism from typical children, and
provided a model for how atypical experience and intrinsic biases might
affect development. This work demonstrated a number of deficits
with traditional gaze analysis techniques and provided novel mechanisms
for interpreting gaze data. |
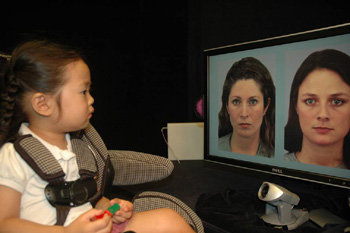 |
Robots as therapeutic devices:
As we have observed in our own clinic and as has been reported by
numerous groups worldwide, many children with autism show increased
motivation, maintain prolonged interest, and even display novel
appropriate social behaviors when interacting with social robots.
Our hope is to exploit this motivation and interest to construct
systems that provide social skills training to supplement the
activities therapists and families already engage in. For
example, in one pilot study, we demonstrated that children engaged in
prosodic training showed the same levels of improvement when using a
robot which provided feedback during part of their therapy time as when
a trained therapist provided the feedback directly throughout the
allotted time. Our therapy work focuses on identifying
the properties of robotic systems that provoke this unique response and
on establishing skill transfer from human-robot pairings to human-human
pairs. |
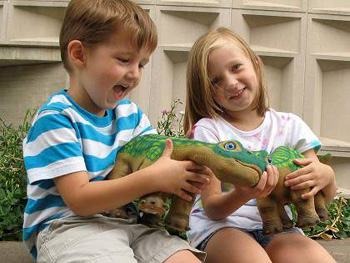 |
Previous Projects:
Cog: Before arriving at Yale, my research focused on the
construction of a humanoid robot named Cog under the direction of Rod
Brooks.
My dissertation work focused on building foundations of a theory of
mind for Cog. This included building systems that represent a "naive
physics" that distinguish animate from inanimate stimuli, an "intuitive
psychology" that attributes intent and goals, and systems that engage
in joint reference such as making eye contact. These systems are based
on models of social skill development in children and models of autism.
This robot was capable of simple imitation of unstructured human arm
movements, and was one of the first attempts to construct behaviors
using a developmental framework inspired by the developmental
progression of skills observed in human infants. |
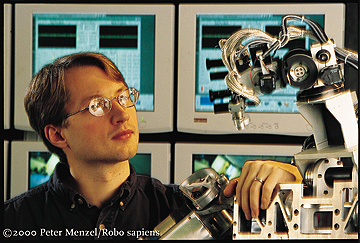 |
Kismet: Kismet
was an exploration of the recognition and generation of social cues.
This robot was capable of generating a variety of social gestures and
facial expressions in response to the perceived social response of a
person. With Cynthia Breazeal,
I constructed systems to exploit social interactions with people in
order to explore just how much a robot can learn when placed in a
supportive learning environment. Kismet was one of the first robots to
excite my interest in using robots as a tool for studying human social
behavior; our work in studying how children reacted to this device
demonstrated that a robot could often elicit detailed explanations from
children on what makes something alive. |
![[Picture of Kismet]](images/kismet.jpg) |